Chinese Irony Detection and Corpus Analysis with BERT
黃靖媛、詹舒宇
Abstract
Irony detection has always been regarded as a difficult task in NLP, due to the contrasting sentiment between the context of an irony sentence and its true meaning. As there has been little research regarding irony detection in Chinese, we designed various training sets based on currently existing Chinese irony corpuses for the task of fine-tuning BERT, and inquired on how different biases affect the model’s training results.
Research Purpose
- Build a BERT model suitable for learning Chinese irony detection, and datasets to be used in fine-tuning
- Taking the biases of the corpus into account, design different training sets and fine-tune the model with each respectively
- Evaluate results and analyze how each bias affects the fine-tuned model’s accuracy
Methodology
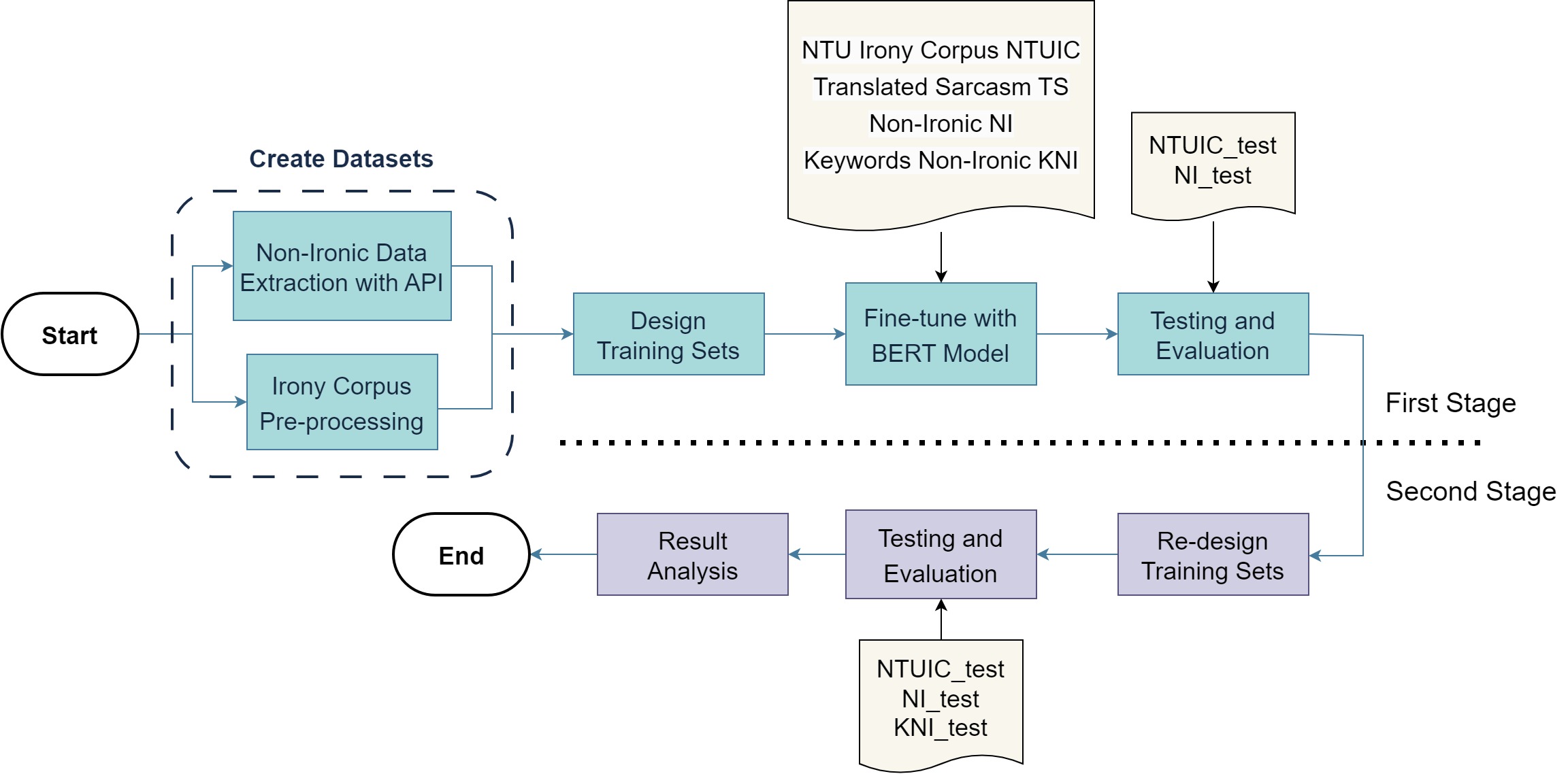
Conclusion and Future Work
From the results of our experiments, we can conclude that out of the two biases, the fixed Chinese irony patterns found in the corpuses affects the fine-tuned model’s performance the most. Adding “irony keywords” can effectively reduce the impact of this bias.
Although there wasn’t a significant improvement with the models fine-tuned by other training sets, rises in evaluation scores could still be observed in most of the models, signifying that the training sets had an overall positive effect on the fine-tuning process.